AI in Drug Discovery
Artificial Intelligence (AI) methods have been shown to be able to design new molecules and to accurately foresee their role in the human body. New drugs will be safer and more effective than they have ever been. Researchers of JKU have won the Tox21 Data Challenge demonstrating that neural AIs can find toxic effects. Günter Klambauer leads an award-winning team of scientists developing AIs that design, improve and assess new drugs. Several AI-generated drugs are currently tested for their ability to inhibit SARS-CoV-2.
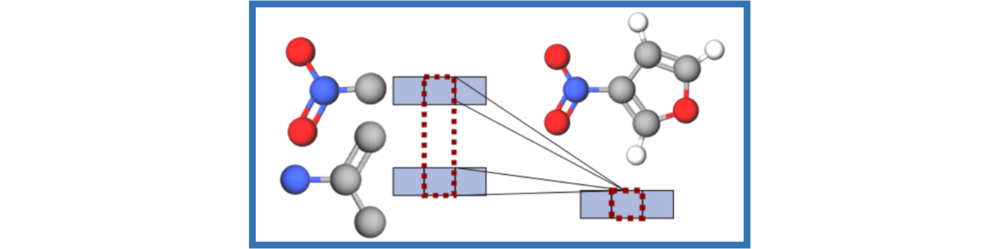
recent publications in AI in Drug Discovery:
- AI4ScienceRobust task-specific adaption of models for drug-target interaction predictionSvensson, E., Hoedt, P., Hochreiter, S., and Klambauer, G.In NeurIPS 2022 AI for Science: Progress and Promises 2022
HyperNetworks have been established as an effective technique to achieve fast adaptation of parameters for neural networks. Recently, HyperNetworks conditioned on descriptors of tasks have improved multi-task generalization in various domains, such as personalized federated learning and neural architecture search. Especially powerful results were achieved in few- and zero-shot settings, attributed to the increased information sharing by the HyperNetwork. With the rise of new diseases fast discovery of drugs is needed which requires proteo-chemometric models that are able to generalize drug-target interaction predictions in low-data scenarios. State-of-the-art methods apply a few fully-connected layers to concatenated learned embeddings of the protein target and drug compound. In this work, we develop a task-conditioned HyperNetwork approach for the problem of predicting drug-target interactions in drug discovery. We show that when model parameters are predicted for the fully-connected layers processing the drug compound embedding, based on the protein target embedding, predictive performance can be improved over previous methods. Two additional components of our architecture, a) switching to L1 loss, and b) integrating a context module for proteins, further boost performance and robustness. On an established benchmark for proteo-chemometrics models, our architecture outperforms previous methods in all settings, including few- and zero-shot settings. In an ablation study, we analyze the importance of each of the components of our HyperNetwork approach.
- ML4MoleculesTask-conditioned modeling of drug-target interactionsSvensson, E., Hoedt, P., Hochreiter, S., and Klambauer, G.In ELLIS Machine Learning for Molecule Discovery Workshop 2022
HyperNetworks have been established as an effective technique to achieve fast adaptation of parameters for neural networks. Recently, HyperNetworks conditioned on descriptors of tasks have improved multi-task generalization in various domains, such as personalized federated learning and neural architecture search. Especially powerful results were achieved in few- and zero-shot settings, attributed to the increased information sharing by the HyperNetwork. With the rise of new diseases fast discovery of drugs is needed which requires proteo-chemometric models that are able to generalize drug-target interaction predictions in low-data scenarios. State-of-the-art methods apply a few fully-connected layers to concatenated learned embeddings of the protein target and drug compound. In this work, we develop a task-conditioned HyperNetwork approach for the problem of predicting drug-target interactions in drug discovery. We show that when model parameters are predicted for the fully-connected layers processing the drug compound embedding, based on the protein target embedding, predictive performance can be improved over previous methods. Two additional components of our architecture, a) switching to L1 loss, and b) integrating a context module for proteins, further boost performance and robustness. On an established benchmark for proteo-chemometrics models, our architecture outperforms previous methods in all settings, including few- and zero-shot settings. In an ablation study, we analyze the importance of each of the components of our HyperNetwork approach.
- JCIMImproving Few- and Zero-Shot Reaction Template Prediction Using Modern Hopfield NetworksSeidl, P., Renz, P., Dyubankova, N., Neves, P., Verhoeven, J., Wegner, J., Segler, M., Hochreiter, S., and Klambauer, G.Journal of Chemical Information and Modeling 2022
Finding synthesis routes for molecules of interest is essential in the discovery of new drugs and materials. To find such routes, computer-assisted synthesis planning (CASP) methods are employed, which rely on a single-step model of chemical reactivity. In this study, we introduce a template-based single-step retrosynthesis model based on Modern Hopfield Networks, which learn an encoding of both molecules and reaction templates in order to predict the relevance of templates for a given molecule. The template representation allows generalization across different reactions and significantly improves the performance of template relevance prediction, especially for templates with few or zero training examples. With inference speed up to orders of magnitude faster than baseline methods, we improve or match the state-of-the-art performance for top-k exact match accuracy for k ≥ 3 in the retrosynthesis benchmark USPTO-50k. Code to reproduce the results is available at github.com/ml-jku/mhn-react.
- QSARBenchmarking recent Deep Learning methods on the extended Tox21 data setSeidl, P., Halmich, C., Mayr, A., Vall, A., Ruch, P., Hochreiter, S., and Klambauer, G.In 2021
The Tox21 data set has evolved into a standard benchmark for computational QSAR methods in toxicology. One limitation of the Tox21 data set is, however, that it only contains twelve toxic assays which strongly restricts its power to distinguish the strength of computational methods. We ameliorate this problem by benchmarking on the extended Tox21 dataset with 68 publicly available assays in order to allow for a better assessment and characterization. The broader range of assays also allows for multi-task approaches, which have been particularly successful as predictive models. Furthermore, previous publications comparing methods on Tox21 did not include recent developments in the field of machine learning, such as graph neural and modern Hopfield networks. Thus we benchmark a set of prominent machine learning methods including those new types of neural networks. The results of the benchmarking study show that the best methods are modern Hopfield networks and multi-task graph neural networks with an average area-under-ROCcurve of 0.91 ± 0.05 (standard deviation across assays), while traditional methods, such as Random Forests fall behind by a substantial margin. Our results of the full benchmark suggest that multi-task learning has a stronger effect on the predictive performance than the choice of the representation of the molecules, such as graph, descriptors, or fingerprints.
- Frontiers in AIThe Promise of AI for DILI PredictionVall, A., Sabnis, Y., Shi, J., Class, R., Hochreiter, S., and Klambauer, G.Frontiers in Artificial Intelligence 2021
Drug-induced liver injury (DILI) is a common reason for the withdrawal of a drug from the market. Early assessment of DILI risk is an essential part of drug development, but it is rendered challenging prior to clinical trials by the complex factors that give rise to liver damage. Artificial intelligence (AI) approaches, particularly those building on machine learning, range from random forests to more recent techniques such as deep learning, and provide tools that can analyze chemical compounds and accurately predict some of their properties based purely on their structure. This article reviews existing AI approaches to predicting DILI and elaborates on the challenges that arise from the as yet limited availability of data. Future directions are discussed focusing on rich data modalities, such as 3D spheroids, and the slow but steady increase in drugs annotated with DILI risk labels.
- arXivModern Hopfield Networks for Few- and Zero-Shot Reaction PredictionarXiv preprint arXiv:2104.03279 2021
An essential step in the discovery of new drugs and materials is the synthesis of a molecule that exists so far only as an idea to test its biological and physical properties. While computer-aided design of virtual molecules has made large progress, computer-assisted synthesis planning (CASP) to realize physical molecules is still in its infancy and lacks a performance level that would enable large-scale molecule discovery. CASP supports the search for multi-step synthesis routes, which is very challenging due to high branching factors in each synthesis step and the hidden rules that govern the reactions. The central and repeatedly applied step in CASP is reaction prediction, for which machine learning methods yield the best performance. We propose a novel reaction prediction approach that uses a deep learning architecture with modern Hopfield networks (MHNs) that is optimized by contrastive learning. An MHN is an associative memory that can store and retrieve chemical reactions in each layer of a deep learning architecture. We show that our MHN contrastive learning approach enables few- and zero-shot learning for reaction prediction which, in contrast to previous methods, can deal with rare, single, or even no training example(s) for a reaction. On a well established benchmark, our MHN approach pushes the state-of-the-art performance up by a large margin as it improves the predictive top-100 accuracy from 0.858±0.004 to 0.959±0.004. This advance might pave the way to large-scale molecule discovery.
- QSAR2021Comparative assessment of interpretability methods of deep activity models for hERGSchimunek, J., Friedrich, L., Kuhn, D., Hochreiter, S., Rippmann, F., and Klambauer, G.2021
Since many highly accurate predictive models for bioactivity and toxicity assays are based on Deep Learning methods, there has been a recent surge of interest in interpretability methods for Deep Learning approaches in drug discovery [1,2]. Interpretability methods are highly desired by human experts to enable them to make design decisions on the molecule based on the activity model. However, it is still unclear which of those interpretability methods are better identifying relevant substructures of molecules. A method comparison is further complicated by the lack of ground truth and appropriate metrics. Here, we present the first comparative study of a set of interpretability methods for Deep Learning models for hERG inhibition. In our work, we compared layer-wise relevance propagation, feature gradients, saliency maps, integrated gradients, occlusion and Shapley values. In the quantitative analysis, known substructures which indicate hERG activity are used as ground truth [3]. Interpretability methods were compared by their ability to rank atoms, which are part of indicative substructures, first. The significantly best performing method is Shapley values with an area under-ROC-curve (AUC) of 0.74 ± 0.12, but also runner-up methods, such as Integrated Gradients, achieved similar results. The results indicate that interpretability methods for deep activity models have the potential to identify new toxicophores. [1] Jiménez-Luna, J., et al. (2020). Nature Machine Intelligence, 2(10), 573-584. [2] Preuer, K., et al. (2019). In Explainable AI: Interpreting, Explaining and Visualizing Deep Learning (pp. 331-345). [3] Czodrowski, P. (2013). Journal of chemical information and modeling, 53, 2240–2251.
- SpringerIndustry-Scale Application and Evaluation of Deep Learning for Drug Target PredictionSturm, N., Mayr, A., Le Van, T., Chupakhin, V., Ceulemans, H., Wegner, J., Golib-Dzib, J., Jeliazkova, N., Vandriessche, Y., Böhm, S., Cima, V., Martinovic, J., Greene, N., Vander Aa, T., Ashby, T., Hochreiter, S., Engkvist, O., Klambauer, G., and Chen, H.2020
Artificial intelligence (AI) is undergoing a revolution thanks to the breakthroughs of machine learning algorithms in computer vision, speech recognition, natural language processing and generative modelling. Recent works on publicly available pharmaceutical data showed that AI methods are highly promising for Drug Target prediction. However, the quality of public data might be different than that of industry data due to different labs reporting measurements, different measurement techniques, fewer samples and less diverse and specialized assays. As part of a European funded project (ExCAPE), that brought together expertise from pharmaceutical industry, machine learning, and high-performance computing, we investigated how well machine learning models obtained from public data can be transferred to internal pharmaceutical industry data. Our results show that machine learning models trained on public data can indeed maintain their predictive power to a large degree when applied to industry data. Moreover, we observed that deep learning derived machine learning models outperformed comparable models, which were trained by other machine learning algorithms, when applied to internal pharmaceutical company datasets. To our knowledge, this is the first large-scale study evaluating the potential of machine learning and especially deep learning directly at the level of industry-scale settings and moreover investigating the transferability of publicly learned target prediction models towards industrial bioactivity prediction pipelines.
- On Failure Modes in Molecule Generation and OptimizationRenz, P., Van Rompaey, D., Wegner, J., Hochreiter, S., and Klambauer, G.2020
There has been a wave of generative models for molecules triggered by advances in the field of Deep Learning. These generative models are often used to optimize chemical compounds towards particular properties or a desired biological activity. The evaluation of generative models remains challenging and suggested performance metrics or scoring functions often do not cover all relevant aspects of drug design projects. In this work, we highlight some unintended failure modes in molecular generation and optimization and how these evade detection by current performance metrics.
- Community Assessment to Advance Computational Prediction of Cancer Drug Combinations in a Pharmacogenomic ScreenMenden, M., Wang, D., Mason, M., Szalai, B., Bulusu, K., Guan, Y., Yu, T., Kang, J., Jeon, M., Wolfinger, R., Nguyen, T., Zaslavskiy, M., Jang, I., Ghazoui, Z., Ahsen, M., Vogel, R., Neto, E., Norman, T., Tang, E., Garnett, M., Veroli, G., Fawell, S., Stolovitzky, G., Guinney, J., Dry, J., and Saez-Rodriguez, J.2019
The effectiveness of most cancer targeted therapies is short-lived. Tumors often develop resistance that might be overcome with drug combinations. However, the number of possible combinations is vast, necessitating data-driven approaches to find optimal patient-specific treatments. Here we report AstraZeneca’s large drug combination dataset, consisting of 11,576 experiments from 910 combinations across 85 molecularly characterized cancer cell lines, and results of a DREAM Challenge to evaluate computational strategies for predicting synergistic drug pairs and biomarkers. 160 teams participated to provide a comprehensive methodological development and benchmarking. Winning methods incorporate prior knowledge of drug-target interactions. Synergy is predicted with an accuracy matching biological replicates for >60% of combinations. However, 20% of drug combinations are poorly predicted by all methods. Genomic rationale for synergy predictions are identified, including ADAM17 inhibitor antagonism when combined with PIK3CB/D inhibition contrasting to synergy when combined with other PI3K-pathway inhibitors in PIK3CA mutant cells.
- Interpretable Deep Learning in Drug DiscoveryPreuer, K., Klambauer, G., Rippmann, F., Hochreiter, S., and Unterthiner, T.2019
Without any means of interpretation, neural networks that predict molecular properties and bioactivities are merely black boxes. We will unravel these black boxes and will demonstrate approaches to understand the learned representations which are hidden inside these models. We show how single neurons can be interpreted as classifiers which determine the presence or absence of pharmacophore- or toxicophore-like structures, thereby generating new insights and relevant knowledge for chemistry, pharmacology and biochemistry. We further discuss how these novel pharmacophores/toxicophores can be determined from the network by identifying the most relevant components of a compound for the prediction of the network. Additionally, we propose a method which can be used to extract new pharmacophores from a model and will show that these extracted structures are consistent with literature findings. We envision that having access to such interpretable knowledge is a crucial aid in the development and design of new pharmaceutically active molecules, and helps to investigate and understand failures and successes of current methods.
- NeurIPSUncertainty Estimation Methods to Support Decision-Making in Early Phases of Drug DiscoveryRenz, P., Hochreiter, S., and Klambauer, G.2019
It takes about a decade to develop a new drug by a process in which a large number of decisions have to be made. Those decisions are critical for the success or failure of a multi-million dollar drug discovery project, which could save many lives or increase life quality. Decisions in early phases of drug discovery, such as the selection of certain series of chemical compounds, are particularly impactful on the success rate. Machine learning models are increasingly used to inform the decision making process by predicting desired effects, undesired effects, such as toxicity, molecular properties, or which wet-lab test to perform next. Thus, accurately quantifying the uncertainties of the models’ outputs is critical, for example, in order to calculate expected utilities, to estimate the risk and the potential gain. In this work, we review, assess and compare recent uncertainty estimation methods with respect to their use in drug discovery projects. We test both, which methods give well calibrated prediction and which ones perform well at misclassification detection. For the latter, we find the entropy of the predictive distribution performs best. Finally, we discuss the problem of defining out-of-distribution samples for prediction tasks on chemical compounds.
- Large-Scale Comparison of Machine Learning Methods for Drug Target Prediction on ChEMBLMayr, A., Klambauer, G., Unterthiner, T., Steijaert, M., Wegner, J., Ceulemans, H., Clevert, D., and Hochreiter, S.2018
Deep learning is currently the most successful machine learning technique in a wide range of application areas and has recently been applied successfully in drug discovery research to predict potential drug targets and to screen for active molecules. However, due to (1) the lack of large-scale studies, (2) the compound series bias that is characteristic of drug discovery datasets and (3) the hyperparameter selection bias that comes with the high number of potential deep learning architectures, it remains unclear whether deep learning can indeed outperform existing computational methods in drug discovery tasks. We therefore assessed the performance of several deep learning methods on a large-scale drug discovery dataset and compared the results with those of other machine learning and target prediction methods. To avoid potential biases from hyperparameter selection or compound series, we used a nested cluster-cross-validation strategy. We found (1) that deep learning methods significantly outperform all competing methods and (2) that the predictive performance of deep learning is in many cases comparable to that of tests performed in wet labs (i.e., in vitro assays).
- DeepSynergy: Predicting Anti-Cancer Drug Synergy with Deep LearningPreuer, K., Lewis, R., Hochreiter, S., Bender, A., Bulusu, K., and Klambauer, G.2018
While drug combination therapies are a well-established concept in cancer treatment, identifying novel synergistic combinations is challenging due to the size of combinatorial space. However, computational approaches have emerged as a time- and cost-efficient way to prioritize combinations to test, based on recently available large-scale combination screening data. Recently, Deep Learning has had an impact in many research areas by achieving new state-of-the-art model performance. However, Deep Learning has not yet been applied to drug synergy prediction, which is the approach we present here, termed DeepSynergy. DeepSynergy uses chemical and genomic information as input information, a normalization strategy to account for input data heterogeneity, and conical layers to model drug synergies.DeepSynergy was compared to other machine learning methods such as Gradient Boosting Machines, Random Forests, Support Vector Machines and Elastic Nets on the largest publicly available synergy dataset with respect to mean squared error. DeepSynergy significantly outperformed the other methods with an improvement of 7.2% over the second best method at the prediction of novel drug combinations within the space of explored drugs and cell lines. At this task, the mean Pearson correlation coefficient between the measured and the predicted values of DeepSynergy was 0.73. Applying DeepSynergy for classification of these novel drug combinations resulted in a high predictive performance of an AUC of 0.90. Furthermore, we found that all compared methods exhibit low predictive performance when extrapolating to unexplored drugs or cell lines, which we suggest is due to limitations in the size and diversity of the dataset. We envision that DeepSynergy could be a valuable tool for selecting novel synergistic drug combinations.DeepSynergy is available via www.bioinf.jku.at/software/DeepSynergy.Supplementary data are available at Bioinformatics online.
- Fréchet ChemNet Distance: A Metric for Generative Models for Molecules in Drug DiscoveryPreuer, K., Renz, P., Unterthiner, T., Hochreiter, S., and Klambauer, G.2018
The new wave of successful generative models in machine learning has increased the interest in deep learning driven de novo drug design. However, method comparison is difficult because of various flaws of the currently employed evaluation metrics. We propose an evaluation metric for generative models called Fréchet ChemNet distance (FCD). The advantage of the FCD over previous metrics is that it can detect whether generated molecules are diverse and have similar chemical and biological properties as real molecules.
- Rectified Factor Networks for Biclustering of Omics DataClevert, D., Unterthiner, T., Povysil, G., and Hochreiter, S.2017
Biclustering has become a major tool for analyzing large datasets given as matrix of samples times features and has been successfully applied in life sciences and e-commerce for drug design and recommender systems, respectively. Factor Analysis for Bicluster Acquisition (FABIA), one of the most successful biclustering methods, is a generative model that represents each bicluster by two sparse membership vectors: one for the samples and one for the features. However, FABIA is restricted to about 20 code units because of the high computational complexity of computing the posterior. Furthermore, code units are sometimes insufficiently decorrelated and sample membership is difficult to determine. We propose to use the recently introduced unsupervised Deep Learning approach Rectified Factor Networks (RFNs) to overcome the drawbacks of existing biclustering methods. RFNs efficiently construct very sparse, non-linear, high-dimensional representations of the input via their posterior means. RFN learning is a generalized alternating minimization algorithm based on the posterior regularization method which enforces non-negative and normalized posterior means. Each code unit represents a bicluster, where samples for which the code unit is active belong to the bicluster and features that have activating weights to the code unit belong to the bicluster.On 400 benchmark datasets and on three gene expression datasets with known clusters, RFN outperformed 13 other biclustering methods including FABIA. On data of the 1000 Genomes Project, RFN could identify DNA segments which indicate, that interbreeding with other hominins starting already before ancestors of modern humans left Africa.https://github.com/bioinf-jku/librfn
- Panelcn.MOPS: Copy-Number Detection in Targeted NGS Panel Data for Clinical DiagnosticsPovysil, G., Tzika, A., Vogt, J., Haunschmid, V., Messiaen, L., Zschocke, J., Klambauer, G., Hochreiter, S., and Wimmer, K.2017
Targeted next-generation-sequencing (NGS) panels have largely replaced Sanger sequencing in clinical diagnostics. They allow for the detection of copy-number variations (CNVs) in addition to single-nucleotide variants and small insertions/deletions. However, existing computational CNV detection methods have shortcomings regarding accuracy, quality control (QC), incidental findings, and user-friendliness. We developed panelcn.MOPS, a novel pipeline for detecting CNVs in targeted NGS panel data. Using data from 180 samples, we compared panelcn.MOPS with five state-of-the-art methods. With panelcn.MOPS leading the field, most methods achieved comparably high accuracy. panelcn.MOPS reliably detected CNVs ranging in size from part of a region of interest (ROI), to whole genes, which may comprise all ROIs investigated in a given sample. The latter is enabled by analyzing reads from all ROIs of the panel, but presenting results exclusively for user-selected genes, thus avoiding incidental findings. Additionally, panelcn.MOPS offers QC criteria not only for samples, but also for individual ROIs within a sample, which increases the confidence in called CNVs. panelcn.MOPS is freely available both as R package and standalone software with graphical user interface that is easy to use for clinical geneticists without any programming experience. panelcn.MOPS combines high sensitivity and specificity with user-friendliness rendering it highly suitable for routine clinical diagnostics.