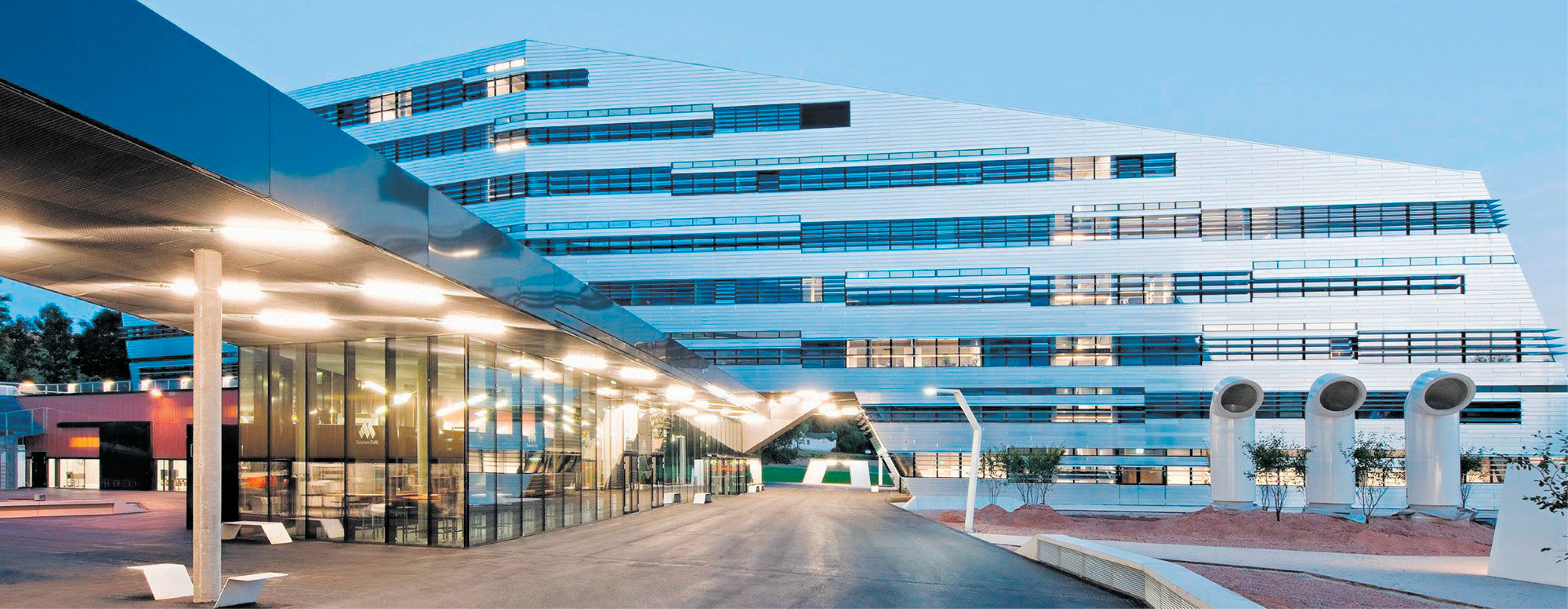
The Institute for Machine Learning conducts research and provides profound education in machine learning. Its research focuses on development of machine learning and statistical methods. We further apply these methods to various domains like medicine, drug discovery, autonomous driving, earth science, natural language processing, control and others. The institute is led by Sepp Hochreiter and is affiliated with Johannes Kepler University Linz and is also one of the founding units of European Lab for Learning and Intelligent Systems (ELLIS).
The Institute for Machine Learning is located in the beautiful city of Linz, Austria. Located near the amazing Austrian alps, the once European capital of culture mixes the right balance between nature and urban life. A part of the institute is also located in the city of Vienna, Austria.
news
Learning Retinal Representations from Multi-modal Imaging via Contrastive Pre-training | Paper accepted with oral talk at MIDL 2023:
Boundary Graph Neural Networks for 3D Simulations | Paper accepted with oral talk at AAAI 2023:
Hopfield Networks is all you need | Paper accepted at ICLR 2021:
link | Talk by Sepp Hochreiter on the topic of Modern Hopfield networks and Dense associative memories:
link | New blog post on the relationship of Performers and Hopfield Networks: